The goal of this project is to upscale images. The images are of shape 640x360 and are converted to be of shape 1280x720. The images are upscaled using a Generative Adversarial Network (GAN). A GAN is an architecture that uses 2 models. The generator model is responsible for mimicking the training data. The discriminator is responsible for deciphering the generated data from the training data. The dataset used is the Berkeley Deep Drive 100k which is comprised of 100,000 images of cars being driven. In a GAN the loss of the generator depends on the discriminator and vice versa. The goal of the generator is to fool the discriminator whilst the discriminator tries not to be fooled. The structure of the generator uses 2 blocks made of deconvolution layers, batch norm, and ReLU. The discriminator uses 3 blocks comprised of a convolutional layer, normalization layer, and lastly the ReLU function is applied to it.
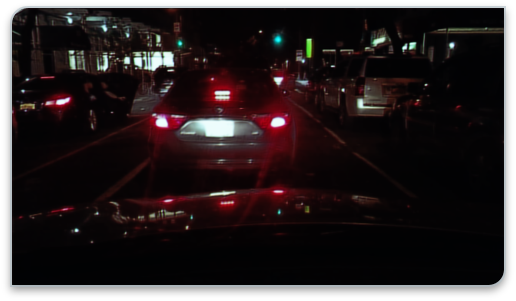
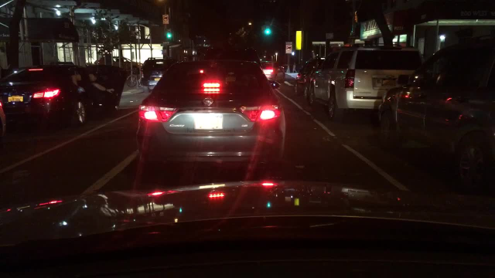